Can DeepFake Detectors Analyze Both Videos and Images? where synthetic media has become increasingly sophisticated, the ability to detect and counter deepfakes has emerged as a critical challenge. Deepfakes, created through the manipulation of audio, video, or images using advanced deep learning techniques, have the potential to spread misinformation, compromise personal privacy, and undermine trust in digital content. As the threat posed by deepfakes continues to grow, the development of effective detection methods capable of analyzing both videos and images has become a top priority for researchers, technology companies, and regulatory bodies alike.
Understanding Deepfakes: A Primer
Before delving into the capabilities of deepfake detectors, it’s essential to understand the nature of deepfakes and the underlying technologies that make their creation possible.
What are Deepfakes?
Deepfakes are a form of synthetic media created using deep learning algorithms, such as generative adversarial networks (GANs) and autoencoders, to manipulate or generate audio, video, or images. These algorithms are trained on vast datasets of multimedia content, allowing them to learn and replicate intricate patterns, facial features, and behaviors with remarkable accuracy.
The term “deepfake” is a combination of the words “deep learning” and “fake,” reflecting the use of deep learning techniques to create highly convincing and realistic fabrications. Deepfakes can be used to superimpose an individual’s face, voice, or gestures onto existing media, creating a seamless and convincing illusion.
Deepfake Creation Process
The process of creating deepfakes typically involves several steps:
- Data Collection: Large datasets of images, videos, or audio recordings are gathered, often from publicly available sources or social media platforms.
- Data Preprocessing: The collected data is preprocessed, cleaned, and organized to prepare it for training the deep learning models.
- Model Training: Deep learning models, such as GANs or autoencoders, are trained on the preprocessed data, allowing them to learn the intricate patterns and features of the target subject or domain.
- Deepfake Generation: Once trained, the models can be used to manipulate or generate synthetic media, blending elements from different sources or creating entirely new fabrications.
- Post-processing and Refinement: The generated deepfake content may undergo additional post-processing and refinement to enhance its realism and believability.
As deepfake technology continues to advance, the quality and sophistication of synthetic media have improved dramatically, making it increasingly difficult to distinguish between genuine and fabricated content.
Potential Risks and Implications
The proliferation of deepfakes poses significant risks and implications across various domains, including:
- Misinformation and Disinformation: Deepfakes can be used to create and spread false narratives, manipulate public opinion, and undermine trust in legitimate sources of information, posing a threat to democratic processes and societal stability.
- Identity Theft and Fraud: By impersonating individuals in audio, video, or image content, deepfakes can facilitate identity theft, financial fraud, and other malicious activities, compromising personal and financial security.
- Revenge Porn and Exploitation: The non-consensual creation and dissemination of explicit deepfake content can lead to the exploitation and harassment of individuals, causing significant emotional distress and reputational damage.
- Erosion of Trust: The widespread distribution of deepfakes can erode public trust in digital media, undermining the credibility of legitimate sources and fostering an environment of skepticism and uncertainty.
To mitigate these risks and preserve the integrity of digital information, the development of effective deepfake detection methods capable of analyzing both videos and images has become a critical priority.
The Role of Deepfake Detectors
Deepfake detectors are computational tools and algorithms designed to identify and distinguish synthetic media from authentic content. These detectors play a crucial role in combating the spread of deepfakes and safeguarding the integrity of digital information across various domains.
Preserving Trust in Digital Media
In our increasingly digital world, where information and content are constantly shared and consumed across various platforms, maintaining trust in the authenticity of digital media is essential. Deepfake detectors help preserve this trust by providing a means to verify the legitimacy of audio, video, and image content, ensuring that we can rely on the information we receive and make informed decisions based on accurate data.
Combating Misinformation and Disinformation
Deepfakes have the potential to be weaponized for the spread of misinformation and disinformation campaigns, which can have far-reaching consequences for individuals, organizations, and societies. Effective deepfake detectors serve as a powerful tool in combating these threats, enabling the timely identification and mitigation of synthetic media before it can cause significant harm.
Protecting Individual Privacy and Security
The non-consensual creation and dissemination of deepfake content, particularly in the form of explicit or compromising media, can severely violate individual privacy and personal security. Deepfake detectors can help identify and remove such content, protecting individuals from exploitation, harassment, and reputational damage.
Safeguarding Democratic Processes and Public Trust
In the political sphere, deepfakes pose a significant threat to democratic processes and public trust. By enabling the detection and removal of fabricated or manipulated media, deepfake detectors can help safeguard the integrity of elections, political discourse, and public decision-making processes, ensuring that citizens have access to accurate and reliable information.
Facilitating Accountability and Legal Recourse
The ability to reliably detect deepfakes is crucial for facilitating accountability and pursuing legal recourse against those who create or distribute synthetic media for malicious purposes. Deepfake detectors can provide valuable evidence and support in investigations and legal proceedings, helping to hold perpetrators accountable for their actions.
Challenges in Deepfake Detection for Videos and Images
While the development of effective deepfake detectors is critical, analyzing both videos and images presents unique challenges that must be addressed to ensure reliable and robust detection capabilities.
Varying Modalities and Data Types
Videos and images represent different modalities and data types, each with its own unique characteristics and challenges. Videos involve temporal information and motion, while images are static representations. Developing detection methods that can effectively handle both modalities requires sophisticated techniques capable of capturing and analyzing the relevant features and artifacts in each data type.
Computational Complexity and Scalability
Analyzing videos and high-resolution images can be computationally intensive, especially when dealing with large volumes of data. Ensuring that deepfake detectors are efficient, scalable, and capable of operating in real-time or near real-time is crucial for practical deployment and widespread adoption.
Diversity of Deepfake Techniques
Deepfake creation techniques are constantly evolving, with new methods and algorithms being developed regularly. Deepfake detectors must be capable of generalizing across a wide range of generation techniques, subjects, and scenarios, while minimizing false positives and false negatives.
Adversarial Attacks and Resilience
As deepfake detection methods become more widely known and adopted, there is a risk of adversarial attacks specifically designed to bypass or fool these detection algorithms. Developing resilient detection strategies that can withstand adversarial attacks and maintain robustness is an ongoing area of research and development.
Data Availability and Privacy Concerns
Training effective deepfake detectors often requires access to large datasets of both authentic and synthetic media. However, acquiring and curating such datasets can be challenging due to privacy concerns, data availability limitations, and the potential for biases or imbalances in the data.
Despite these challenges, researchers and developers have made significant strides in developing detection methods capable of analyzing both videos and images, leveraging a variety of techniques and approaches.
Approaches to Deepfake Detection for Videos and Images
Researchers and developers have explored various approaches to deepfake detection, each with its strengths and limitations when it comes to analyzing videos and images. Here are some of the most promising techniques:
Traditional Media Forensics
Traditional media forensics techniques, such as pixel-level analysis, compression artifact analysis, and metadata examination, can be applied to detect inconsistencies or anomalies in synthetic media. These methods can be effective for certain types of deepfakes, particularly those involving image manipulation or basic video editing techniques.
However, traditional forensics methods may be limited in their ability to detect more sophisticated and high-quality synthetic content, as deepfake algorithms continue to improve and learn to mimic authentic media more closely.
Deep Learning-based Detection
Deep learning algorithms, particularly convolutional neural networks (CNNs) and recurrent neural networks (RNNs), have shown promising results in detecting deepfakes in both videos and images. These models are trained on large datasets of authentic and synthetic media, learning to identify subtle patterns and artifacts that distinguish deepfakes from genuine content.
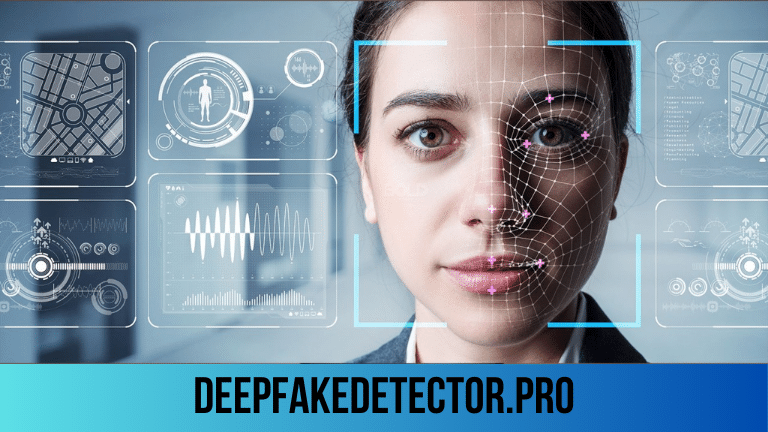
FAQs
1. Can DeepFake detectors analyze both videos and images?
Yes, DeepFake detectors are designed to analyze both videos and images. They utilize various algorithms and techniques to identify signs of manipulation, such as inconsistencies in facial movements, lighting, shadows, and other anomalies that may indicate tampering.
2. How do DeepFake detectors work on videos compared to images?
DeepFake detectors for videos analyze sequences of frames to identify temporal inconsistencies and unnatural movements, such as mismatched lip-syncing or irregular blinking patterns. For images, detectors focus on spatial anomalies, such as unnatural blending of facial features or inconsistent lighting and shadows, which can indicate digital manipulation.
3. Are DeepFake detectors equally effective for videos and images?
While DeepFake detectors are effective for both media types, their effectiveness can vary based on the complexity of the DeepFake. Videos provide more data points due to multiple frames, allowing detectors to analyze motion and temporal patterns, which can be more challenging to fake consistently. Images, on the other hand, rely solely on spatial analysis, which can be more straightforward but also easier for sophisticated fakes to bypass.
4. What technologies are used in DeepFake detectors for analyzing videos and images?
DeepFake detectors use a combination of machine learning, computer vision, and artificial intelligence techniques. For videos, these technologies analyze motion patterns, facial expressions, and audio-visual synchronization. For images, they detect anomalies in texture, lighting, and feature alignment. Neural networks, particularly convolutional neural networks (CNNs) and recurrent neural networks (RNNs), are commonly employed in these processes.
5. Can the same DeepFake detector be used for both videos and images, or are separate tools needed?
Many DeepFake detection tools are versatile and can analyze both videos and images using the same underlying technology, although some specialized tools may be optimized for one type over the other. The core principles of detecting inconsistencies and anomalies are similar, but the specific algorithms and techniques may differ slightly to account for the unique characteristics of videos and images.