Are DeepFake Detectors 100% accurate? highly realistic synthetic media that can manipulate audio, video, or images to depict events that never occurred. These fabricated content pieces pose a significant threat to individual privacy, societal trust, and the integrity of information sources. As a countermeasure, researchers and technology companies have developed various deepfake detection methods, collectively known as deepfake detectors. However, the question remains: Are these detectors 100% accurate in identifying deepfakes? This article delves into the intricate world of deepfake detection, exploring its challenges, limitations, and the ongoing battle against this evolving technology.
The Rise of Deepfakes
Before examining the accuracy of deepfake detectors, it is essential to understand the phenomenon of deepfakes and their potential impact. Deepfakes leverage advanced generative adversarial networks (GANs) and other deep learning techniques to create highly convincing synthetic media. These AI-generated content pieces can superimpose an individual’s face onto another person’s body, alter facial expressions, or even generate entirely new personas from scratch.
The initial emergence of deepfakes was primarily associated with non-consensual pornography, where individuals’ faces were superimposed onto explicit videos, causing significant harm and reputational damage. However, the implications of deepfakes extend far beyond this nefarious application. Deepfakes can be weaponized for disinformation campaigns, financial fraud, and even geopolitical manipulation, posing a severe threat to democratic processes, national security, and societal stability.
The Need for Deepfake Detection
As the proliferation of deepfakes escalates, the need for effective detection mechanisms has become paramount. Failing to identify and counteract deepfakes can have severe consequences, ranging from personal and reputational harm to the erosion of public trust in institutions and the media. Deepfake detection techniques aim to analyze and scrutinize digital media for telltale signs of manipulation, enabling the identification and flagging of potential deepfakes.
Conventional Approaches to Deepfake Detection
Early efforts in deepfake detection relied on traditional computer vision and signal processing techniques. These approaches focused on analyzing visual artifacts, inconsistencies in lighting or shadows, unnatural facial movements, and other anomalies that could indicate manipulation. However, as deepfake generation techniques became more sophisticated, these conventional methods proved increasingly inadequate, often failing to detect cutting-edge deepfakes.
Deep Learning-Based Deepfake Detectors
To combat the ever-evolving deepfake landscape, researchers turned to deep learning and AI-powered solutions. These advanced deepfake detectors leverage convolutional neural networks (CNNs) and other deep learning architectures to learn and recognize patterns and anomalies indicative of synthetic media. By training on vast datasets of authentic and deepfake media, these models can identify subtle inconsistencies and artifacts that may elude human perception.
Some of the commonly used deep learning-based deepfake detection techniques include:
- CNN-based Detectors: Convolutional neural networks are trained to analyze visual features and identify discrepancies in facial landmarks, textures, and other visual cues that may signify manipulation.
- Attention-based Detectors: These models employ attention mechanisms to focus on specific regions of interest within an image or video, such as facial features or inconsistencies in background elements, to improve detection accuracy.
- Temporal Detectors: For video deepfakes, temporal detectors analyze the temporal dynamics of facial movements, lip-sync inconsistencies, and unnatural transitions between frames, which can be indicative of manipulation.
- Ensemble Detectors: By combining multiple detection models, ensemble detectors leverage the strengths of various approaches, potentially improving overall detection performance.
Challenges and Limitations of Deepfake Detectors
While deep learning-based deepfake detectors have demonstrated promising results, they are not without their challenges and limitations. Several factors contribute to the potential inaccuracies and shortcomings of these detection methods.
- Adversarial Attacks and Deepfake Evolution
One of the primary challenges in deepfake detection is the constant evolution of deepfake generation techniques. As detectors become more sophisticated, deepfake creators adapt their methods to evade detection. This cat-and-mouse game between detection and generation leads to a continuous arms race, where detectors must constantly be updated and retrained to keep pace with the latest deepfake techniques.
Furthermore, deepfake creators can employ adversarial attacks, deliberately crafting deepfakes to fool detection models. By introducing carefully designed perturbations or noise into the synthetic media, adversarial attacks can exploit the weaknesses of detectors, leading to false negatives (failing to detect a deepfake).
- Data Limitations and Bias
The performance of deep learning-based detectors heavily relies on the quality and diversity of the training data. Obtaining a large, representative dataset of authentic and deepfake media can be challenging, especially as deepfake techniques evolve. Moreover, biases in the training data, such as overrepresentation of certain demographics or facial features, can lead to inaccuracies and unfair treatment of underrepresented groups.
- Computational Constraints and Scalability
Deploying and running deep learning-based deepfake detectors at scale can be computationally intensive, particularly for real-time applications or large-scale media analysis. This limitation may hinder the adoption of advanced detectors in resource-constrained environments or for applications requiring immediate detection.
- Generalization and Cross-Domain Challenges
Many deepfake detectors are trained on specific types of media or deepfake generation techniques. However, their performance may degrade when applied to unseen or cross-domain scenarios, such as deepfakes generated using different techniques or involving different types of media (e.g., audio deepfakes).
- Interpretability and Explainability
While deep learning models can achieve high detection accuracy, they often lack transparency and interpretability. Understanding the reasoning behind a model’s decisions and the specific features or artifacts it relies on for detection can be challenging, which may hinder trust and adoption in critical applications.
Ongoing Research and Future Directions
Given the challenges and limitations of current deepfake detection methods, ongoing research efforts aim to address these issues and improve the accuracy and robustness of detectors. Some promising research directions include:
- Adversarial Training and Robust Detectors
Researchers are exploring adversarial training techniques to improve the robustness of detectors against adversarial attacks. By incorporating adversarial examples during the training process, detectors can learn to recognize and mitigate the effects of these attacks, potentially improving their accuracy and resilience.
- Multimodal Detection and Fusion
Rather than relying solely on visual cues, multimodal detection approaches combine information from multiple modalities, such as audio, text, and contextual metadata. By fusing these complementary sources of information, multimodal detectors can leverage a broader range of signals to enhance detection accuracy.
- Unsupervised and Self-Supervised Learning
To overcome the limitations of labeled data availability, researchers are investigating unsupervised and self-supervised learning techniques for deepfake detection. These methods aim to learn the underlying patterns and distributions of authentic media without relying on extensive labeled datasets, potentially enabling more generalizable and scalable detectors.
- Interpretable and Explainable Models
Improving the interpretability and explainability of deepfake detection models is another area of active research. By developing models that can provide human-understandable explanations for their decisions, trust and adoption in critical applications can be facilitated, while also enabling better debugging and refinement of detection algorithms.
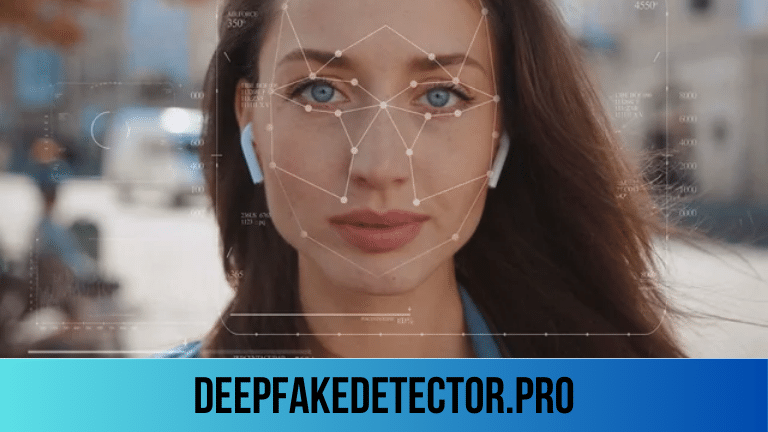
- Collaborative Efforts and Standardization
Given the global nature of the deepfake threat, collaborative efforts among researchers, industry, and policymakers are crucial for advancing deepfake detection capabilities. Standardization of benchmarking datasets, evaluation metrics, and best practices can further accelerate progress in this field.
Conclusion
The rise of deepfakes poses a significant challenge to the integrity of digital media and the trust in information sources. While deepfake detection techniques, particularly those based on deep learning, have made substantial progress, they are not yet 100% accurate. The ongoing arms race between deepfake generation and detection, coupled with various technical and practical limitations, highlights the need for continued research and innovation in this domain.
Achieving near-perfect accuracy in deepfake detection may prove elusive, as the sophistication of deepfake generation techniques continues to evolve. However, by addressing the challenges of adversarial attacks, data limitations, computational constraints, generalization, and interpretability, researchers can strive to develop more robust, accurate, and trustworthy deepfake detectors.
Ultimately, the battle against deepfakes requires a multifaceted approach involving technological advancements, legal and regulatory frameworks, media literacy campaigns, and collaboration among stakeholders. While deepfake detectors play.